Text-to-image
An alternative way to text-to-video generation is text-to-image-to-video generation. I tested this method in hope that it could give me more control of the generated video in terms of quality and visual style.
To add style to the image, I tried 1) adding the style through prompting; 2) fine-tuning technique such as DreamBooth.
Through the tests, I found out that DreamBooth is not. the best training technique to add style since it is more subject/object focused. Nevertheless, both the prompting technique and DreamBooth customized training yield satisfying styled images.
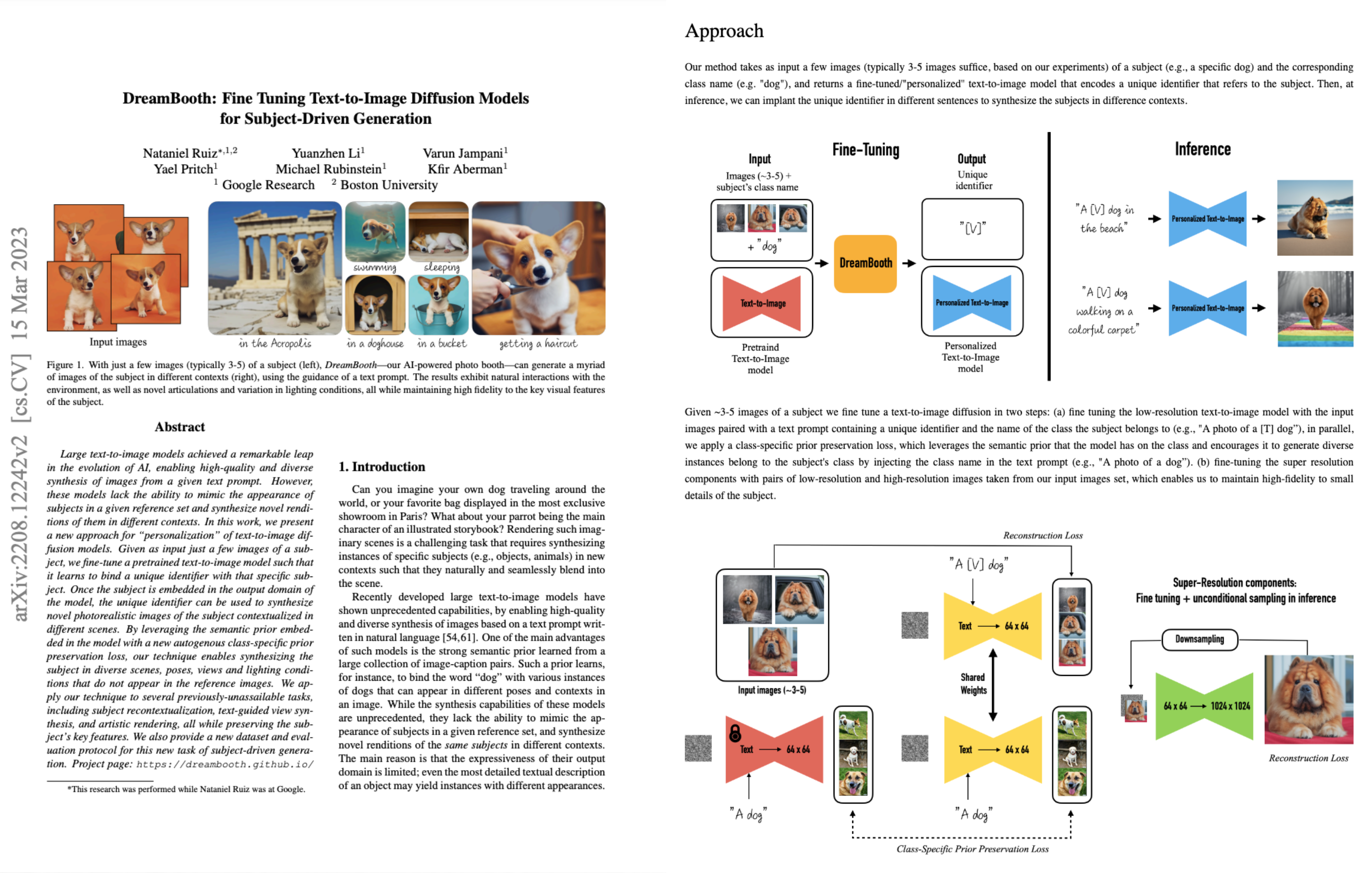
DreamBooth paper
DreamBooth training
Results
Prompt:
Imagine a first-person perspective scene where you are sitting in a room. In the far top left of your view, there's a window through which a cicada can be seen in the distance, perched on a tree branch outside. Close to you in the bottom right, there's a table fan, its blades slightly blurred as they spin. Directly ahead in the center, at a distance, is a TV on a stand, displaying a vibrant, colorful show. The room is softly lit, creating a cozy, relaxed atmosphere.
Prompt:
A landscape with a river in the bottom left corner, surrounded by lush greenery, and a small airplane with blue and white colors in the top right corner against a backdrop of an orange and purple sky at dawn or dusk.
Prompt:
Cicada in background left, fan in foreground right, and TV in foreground middle.
Adding style via prompt
Alternatively, I tried to style the image directly through the prompt. The styling part of the text prompt of this set of images were added onto the LTU-generated scene description by GPT-4. The image generation model used was stable-diffusion-xl-base-1.0. The results were consistent, accurate and pleasing.
β
Prompt:
An image of fountain far in the middle, violin music close on the right, children chattering far on the left, detailed, realistic, in style of Amy Friend, Man Ray, Kurt Schwitter.
With or without styling?
Since I was impressed by the diffusion model, I started to wonder if it is even necessary to style the image generation. To find out, I compared the generated images with and without styling side by side.
Prompt:
A person is walking down the street, and as they walk, they hear the sound of footsteps. Suddenly, a car honks its horn, and the person stops to look around for traffic.
Prompt:
The scene of a person walking on snowy ground while carrying an object that makes noise.
Without directed styling, diffusion model took the liberty of adding one, and it is hard to know beforehand what style it would choose. Β While the customized Dreambooth training may limit the imagination, it did give a more consistent outcome. So I decided to move forward with the diffusion model customized with Dreambooth training.